Earlier this week, we took a look at last season’s most and least consistent fantasy players (by both production and usage). Sometimes that’s just due to luck or variance, but other times it’s due to the specific nature of a player’s role. For instance, wide receivers heavily reliant on deep targets for fantasy production are far more volatile than, say, a true possession wide receiver like Cole Beasley. In today’s article I wanted to focus on another oft-forgotten component to fantasy volatility — gamescript.
In each chart below, broken down by position, we’re looking at how often a player’s team was trailing or leading and each player’s fantasy splits in both categories. The difference between these numbers represents how much a player benefited from a specific gamescript (in this case, either leading or trailing). The more “gamescript dependent” or “gamescript sensitive” players will be the ones on either end of the chart.
By determining how gamescript dependent a player was last season, we should be able to make some educated guesses about their role next season. If a team’s quarterback scored a high percentage of his fantasy points when trailing last season, and the inverse was true for the running back, we might surmise that the team “played it safe” when ahead in games. This might mean that if this team had a game against the Chiefs (i.e. they’re unlikely to be leading), that quarterback might be an interesting and underpriced DFS play that week. Today, we’ll be taking a look at every fantasy-relevant position but focusing mostly on quarterbacks and running backs.
Quarterback
As evident in the chart above, quarterbacks are typically far more productive when trailing. This makes sense intuitively — when there’s more of an urgency to put points on the board, teams will run less and throw more. Last season, on average, teams passed 65.0% of the time when trailing, but only 49.8% of the time when leading. And teams will also run at a quicker pace when trailing, which means more total plays and more total opportunities for fantasy points. For instance, last season the Cowboys averaged 30.1 seconds per play when leading by seven or more points but 21.0 seconds per play when trailing by seven or more points.
If there’s an exception to this rule with quarterbacks — and it seems at least partially evident by the chart above — it’s that dual-threat quarterbacks might actually benefit from positive gamescripts (i.e. playing from ahead). I think the relationship here is that winning/leading in a game is heavily correlated to points being scored, which is heavily correlated to number of red-zone drives. And the more red-zone drives, the more opportunities for goal-line vulturing.
Baker Mayfield was our most gamescript dependent quarterback in 2020, and I think that should not be a surprise at all. Cleveland called a pass on only 42% of their plays when leading last season (fifth-fewest), and how could you blame them? Their offensive line had the league’s best run blocking grade, per PFF, and they have an all-time great running back tandem in Nick Chubb and Kareem Hunt. I suppose Mayfield could be a redraft sleeper if we expect Cleveland’s defense to decline, but I don’t see that happening. Instead, if I have any exposure to Mayfield this year, I imagine it will only come via DFS in weeks when he’s an underpriced underdog in a plus matchup.
Carson Wentz, Kirk Cousins, Drew Brees, Deshaun Watson, Derek Carr, Patrick Mahomes, Russell Wilson, and Philip Rivers also had splits that implied they were used as sort of Alex Smith-esque game managers in 2020. However, this was only backed up by usage in the cases of Cousins, Brees, Carr, and Rivers, who all ranked bottom-12 in pass percentage when leading. I’d certainly expect this trend to continue for Cousins and Carr at least, as this is the third-consecutive season they’ve both ranked top-six in this stat. Like with Mayfield, Cousins and Carr should be avoided for DFS in weeks they’re heavily favored but prioritized in games they enter as underdogs.
Running Backs 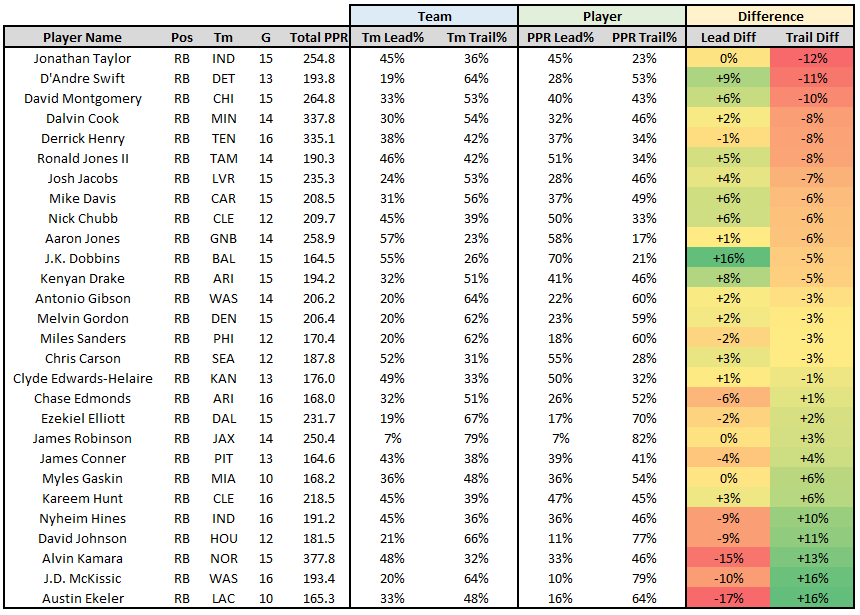
While all other positions benefited from negative gamescript, the opposite was true for the running back position. Remember, when teams are playing with a lead, they’re more likely to run the ball.
The running backs who were most hurt by negative gamescript were Jonathan Taylor, D’Andre Swift, David Montgomery, Dalvin Cook, Derrick Henry, Ronald Jones, and then Josh Jacobs. The running backs who most benefited from positive gamescript were J.K. Dobbins, D’Andre Swift, Kenyan Drake, David Montgomery, and Nick Chubb.
Seeing Swift and Montgomery here was a slight surprise, but for the most part I think these lists make a lot of sense in hindsight. For instance, Chubb saw 33 fewer targets than Kareem Hunt. Taylor saw 37 fewer targets than Nyheim Hines. Which is to say they were not bell cows (defined: here) — they played the workhorse role in a committee with the other running back serving as something more closely approximating the scatback role. So, when their team was trailing (teams run more frequently when leading) they were routinely pulled for the more capable pass-catching back. When the team was leading, they’d go run-heavy and let their early-down workhorse dominate touches (especially near the goal-line) and take time off the clock.
Derrick Henry wasn’t in a committee, but he was also a non-factor in the passing game (1.2 receptions per game). Uncoincidentally, he averaged 12.8 more FPG in victories (24.8) than losses (12.0) last season. Josh Jacobs wasn’t far behind Henry, averaging 11.0 more FPG in victories (21.3) than losses (10.3). And that’s been true for him throughout his whole career — averaging 20.9 FPG in victories as opposed to just 10.1 FPG in losses.
One of the biggest questions of the offseason is whether or not Taylor will be a bell cow in 2021. As a worst-case scenario he’s something approximating (a rich man’s) Marlon Mack, an all-time gamescript sensitive running back. Across his career, Mack averages 21.1 FPG in games Indianapolis won by 14 or more points, but just 9.3 FPG in all other games. As a best-case scenario, Taylor is a gamescript-proof bell cow heavily utilized as both a runner and a pass-catcher. If he’s a bell cow, I wouldn’t be surprised if he comes close to leading the position in fantasy points. But, if Nyheim Hines resumes his 2020 role (or worse yet, if Marlon Mack also eats into playing time), Taylor is seriously overpriced at current ADP (RB6).
On the opposite end of the spectrum, the following running backs benefited most from negative gamescript (and were most at a detriment under positive gamescript): Austin Ekeler, J.D. McKissic, Alvin Kamara, David Johnson, Nyheim Hines, Kareem Hunt, Myles Gaskin, and Chase Edmonds. Again, these running backs were their teams’ primary pass-catching backs. I think the important stat here is that, in PPR leagues, a target is worth 2.73 times as much as a carry for fantasy running backs. So, although Kamara is likely to see fewer red zone opportunities in games New Orleans is trailing, he can at least offset that by an increase in targets. Last season, he averaged 23.1 FPG and 6.2 targets per game in victories, but 31.0 FPG and 9.8 targets per game in losses.
Although DFS players like to target running backs likely to win their games, and while that correlation is usually correct, it depends on the specific running back and their role. For instance, as the data here suggests, I’d be more inclined to play a pass-catching specialist in a game they’re likely to be trailing throughout.
Wide Receivers
Like with quarterbacks, you’ll see all wide receivers benefited greatly when their team was trailing. This makes sense considering how heavily a wide receiver’s production is tied to their quarterback. Overall, I don’t see much significance in these numbers, at least in terms of looking for a serious takeaway for 2021 drafts.
However, I will say, for the most part, players who ranked highly in red-zone target market share also ranked highly in differential when leading. The inverse was also mostly true, and that should make sense intuitively — teams outscoring their opponents are more likely to reach the red zone (and then end zone) than teams already trailing, and so touchdown potential will be higher for these types of receivers when leading. Intuitively, it might also make sense that deep threats performed better when trailing, when there’s more of an urgency to put points on the board, and more of a demand for big plays. However, the data didn’t really bear this out.
Tight Ends
With tight ends, we see a similar trend to wide receivers — an increase in scoring when trailing. We also see, like with wide receivers, a number of these players posted similar splits to their quarterbacks. However, and again, like with wide receivers, I’m struggling to find any serious fantasy implications.